반응형
### Basic Function ###
import math
import numpy as np
import matplotlib.pyplot as plt
plt.style.use(['seaborn-whitegrid'])
### A LINEAR FUNCTION ###
# y = ax + b
# a: 기울기, b: y절편
# 그래프 상에서 직선인 그래프(linear)
def linear_function(x):
a = 0.5
b = 2
return a * x + b
print(linear_function(5)) # 4.5
x = np.arange(-5, 5, 0.1)
y = linear_function(x)
plt.plot(x, y)
plt.xlabel('x')
plt.ylabel('y')
plt.title('LINEAR FUNCTION')
plt.show()
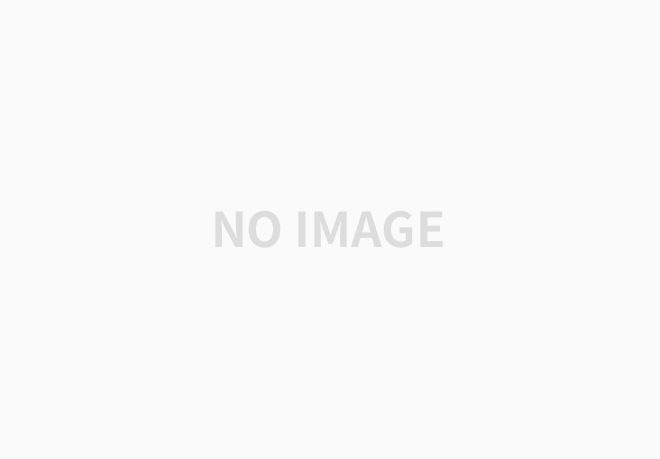
### QUADRATIC FUNCTION ###
# y = ax^2 + bx + c
# 일반적으로 두 개의 실근을 가짐
def quadratic_function(x):
a = 1
b = -1
c = -2
return a*x**2 + b*x + c
print(quadratic_function(2)) # 0
x = np.arange(-5, 5, 0.1)
y = quadratic_function(x)
plt.plot(x, y)
plt.xlabel('x')
plt.ylabel('y')
plt.title('QUADRATIC FUNCTION')
# plt.show()
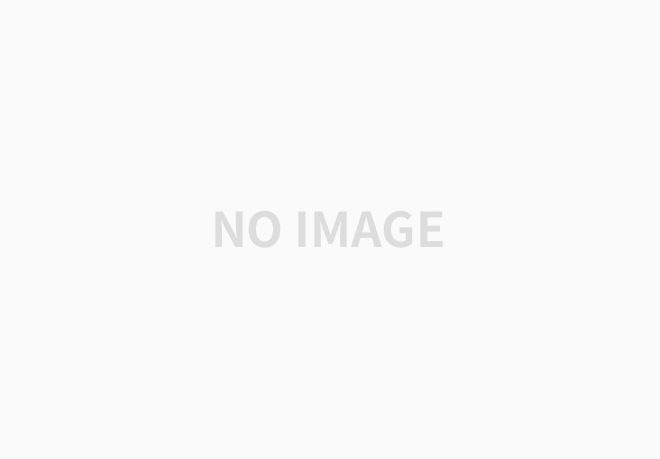
### CUBIC FUNCTION (polynomial function) ###
# y = a*x^3 + b*x^2 + c*x + d
def cubic_function(x):
a = 4
b = 0
c = -1
d = -8
return a*x**3 + b*x**2 + c*x + d
print(cubic_function(3)) # 97
x = np.arange(-5, 5, 0.1)
y = cubic_function(x)
plt.plot(x, y)
plt.xlabel('x')
plt.ylabel('y')
plt.title('CUBIC FUNCTION')
plt.show()
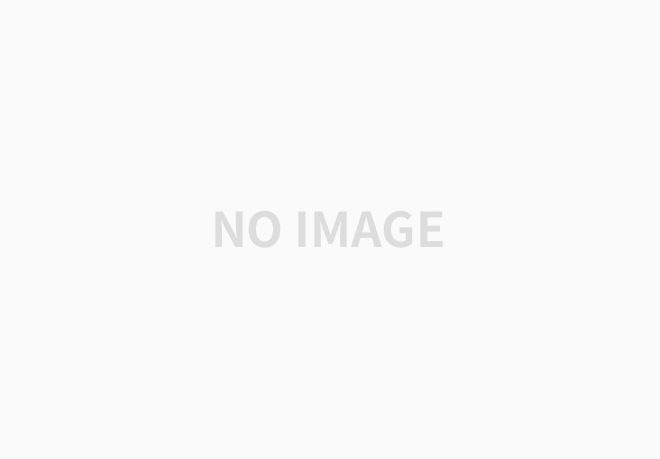
### MIN & MAX VALUES OF A FUNCTION ###
def my_func(x):
a = 1
b = -3
c = 10
return a*x**2 + b*x + c
x = np.arange(-10, 10, 0.1)
y = my_func(x)
plt.plot(x, y)
plt.xlabel('x')
plt.ylabel('y')
plt.scatter(1.5, my_func(1.5))
plt.text(1.5-1.5, my_func(1.5)+10, 'min value of f(x)\n({}, {})'.format(1.5, my_func(1.5)), fontsize=10)
plt.title('MY FUNC')
plt.show()
min_val = min(y)
print(min_val)
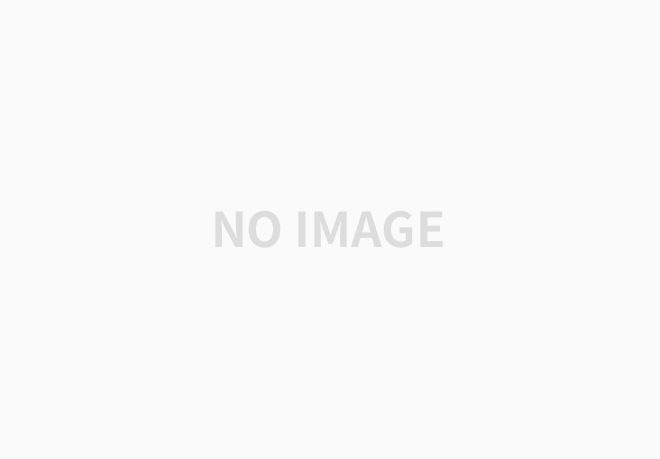
반응형
'Deep Learning' 카테고리의 다른 글
Reinforcement 'CartPole-v1' (0) | 2022.09.23 |
---|---|
Reinforcement Learning (0) | 2022.09.19 |
Linear Models_MLBasic.03 (0) | 2022.09.16 |
ScikitLearn_MLBasic.02 (0) | 2022.09.16 |
Machine Learning_MLBasic.01 (0) | 2022.09.16 |